Risk is an inherent part of every business operation. Traditionally, risk management has been largely reactive, focusing on mitigating threats after they materialize. However, the advent of big data and advanced analytics has ushered in a new era of proactive risk management. Predictive analytics, a subset of data analytics, empowers organizations to anticipate risks by analyzing historical and real-time data to identify patterns and trends. The transition from reactive to proactive risk mitigation is reshaping the business landscape.
Predictive analytics helps identify and address potential risks proactively. This proactive approach not only safeguards assets and reputation but also drives strategic decision-making. In the following sections, we will delve into the intricacies of predictive analytics and explore how it can be applied to various risk management scenarios.
Understanding Predictive Analytics
Predictive analytics is a subset of data analytics that employs statistical algorithms and machine learning techniques to forecast future events based on historical and present data. Essentially, it involves identifying patterns within data to predict potential outcomes. This powerful tool goes beyond merely describing what happened in the past; it delves into predicting what might happen in the future.
In the realm of risk management, predictive analytics serves as a cornerstone. By analyzing past incidents, market trends, and other relevant data, organizations can identify potential risks before they materialize. This proactive approach allows for the development of effective mitigation strategies, safeguarding assets, and ensuring business continuity.
From financial institutions assessing credit risk to healthcare providers predicting disease outbreaks, predictive analytics is reshaping the way organizations approach uncertainty. By harnessing the power of data, businesses can make more informed decisions, optimize operations, and gain a competitive edge.
Data Collection and Preparation
The adage “garbage in, garbage out” holds true in the realm of predictive analytics. The quality of data directly impacts the accuracy and reliability of predictions. Therefore, meticulous data collection and preparation are paramount.
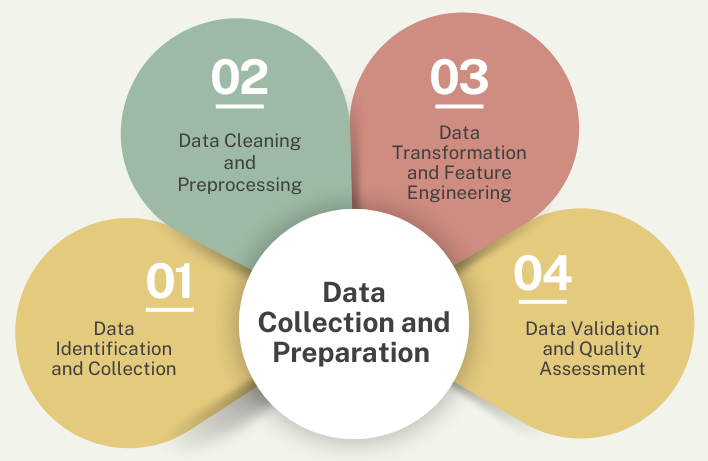
Data Identification and Collection
The first step involves identifying relevant data sources. These can include internal systems, external databases, and third-party data providers. It’s crucial to ensure data consistency, completeness, and relevance to the specific risk being analyzed.
Data Cleaning and Preprocessing
Raw data often contains inconsistencies, errors, and missing values. Data cleaning involves handling these issues to create a clean and usable dataset. This process includes removing duplicates, correcting errors, and imputing missing values.
Data Transformation and Feature Engineering
Raw data often needs to be transformed into a suitable format for predictive modeling. Feature engineering is crucial for optimizing model performance. By crafting relevant features from raw data, we empower models to identify patterns and make accurate predictions.
Data Validation and Quality Assessment
Before feeding data into predictive models, it’s essential to validate its quality. This involves checking for data consistency, accuracy, and completeness. By ensuring data quality, organizations can increase the confidence in the predictions generated by the model.
In conclusion, the quality of data is the cornerstone of successful predictive analytics. Investing time and resources in data collection, cleaning, and preparation is crucial for deriving accurate and actionable insights.
Predictive Modeling Techniques
Predictive modeling is the heart of risk management. It involves selecting the appropriate model based on the nature of the data and the specific risk being assessed. Several techniques have proven effective in various risk management scenarios.
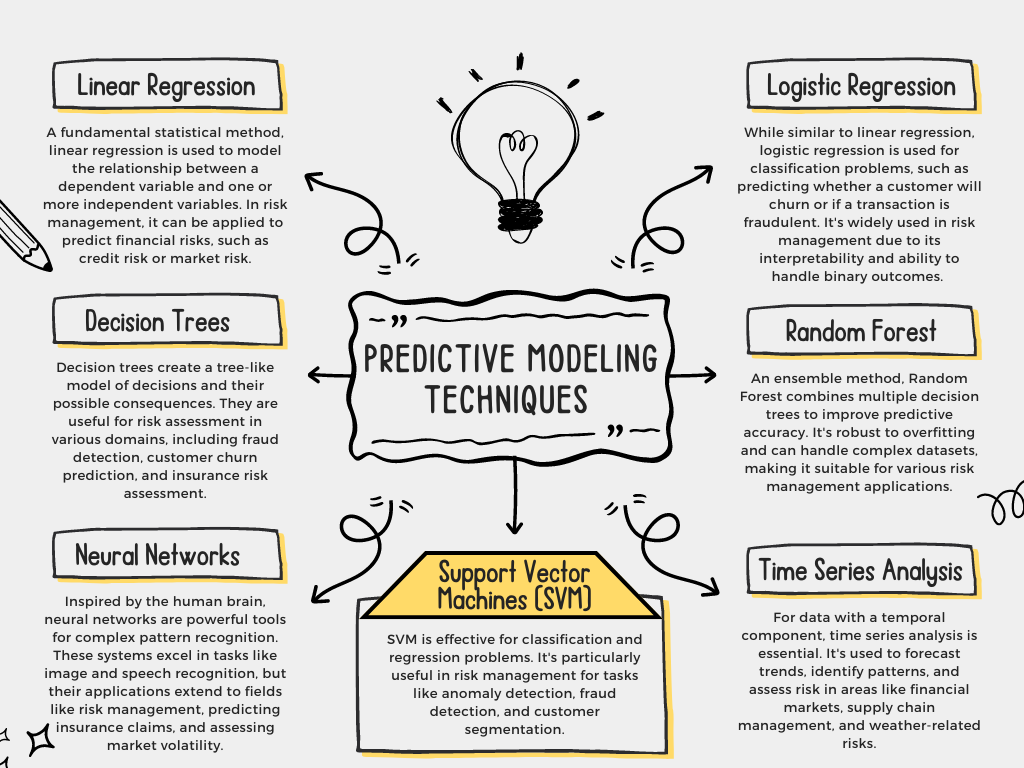
Choosing the Right Model
Selecting the appropriate predictive model depends on several factors, including the type of data, the desired outcome, and the complexity of the problem. Often, a combination of models can be used to create more accurate and robust predictions. It’s essential to evaluate model performance using appropriate metrics and continuously refine the models based on new data and insights.
By understanding these techniques and their applications, organizations can effectively leverage predictive analytics to identify, assess, and mitigate risks.
Risk Identification and Assessment
Predictive analytics empowers organizations to move beyond reactive risk management by proactively identifying potential threats. By analyzing historical and real-time data, businesses can uncover patterns and trends indicative of future risks.
Identifying Potential Risks
- Anomaly Detection: Predictive models can identify unusual patterns in data that deviate from established norms. These anomalies can signal potential risks such as fraud, equipment failure, or supply chain disruptions.
- Scenario Analysis: By simulating various potential outcomes, scenario modelling empowers organizations to make informed decisions, allocate resources effectively, and develop robust contingency plans. This proactive approach to risk management enables businesses to navigate uncertainties with greater confidence.
- Early Warning Systems: Predictive models can serve as early warning systems by forecasting the likelihood of specific events, such as natural disasters, economic downturns, or cyberattacks. This allows businesses to prepare contingency plans and minimize potential losses.
Risk Assessment and Prioritization
By quantifying risk impact and likelihood, organizations can prioritize mitigation efforts effectively. This involves:
- Risk Scoring: Quantifying risks through numerical scoring based on their potential impact and probability.
- Risk Ranking: Prioritizing risks based on their overall score to focus mitigation efforts on the most critical threats.
- Portfolio Risk Assessment: Evaluating the aggregate risk exposure across different business units or portfolios.
By assessing risk impact and probability, organizations can strategically allocate resources and implement effective countermeasures.
Risk Mitigation Strategies
Developing and implementing effective mitigation strategies is crucial after identifying and assessing potential risks. Predictive analytics offers valuable insights to inform this process.
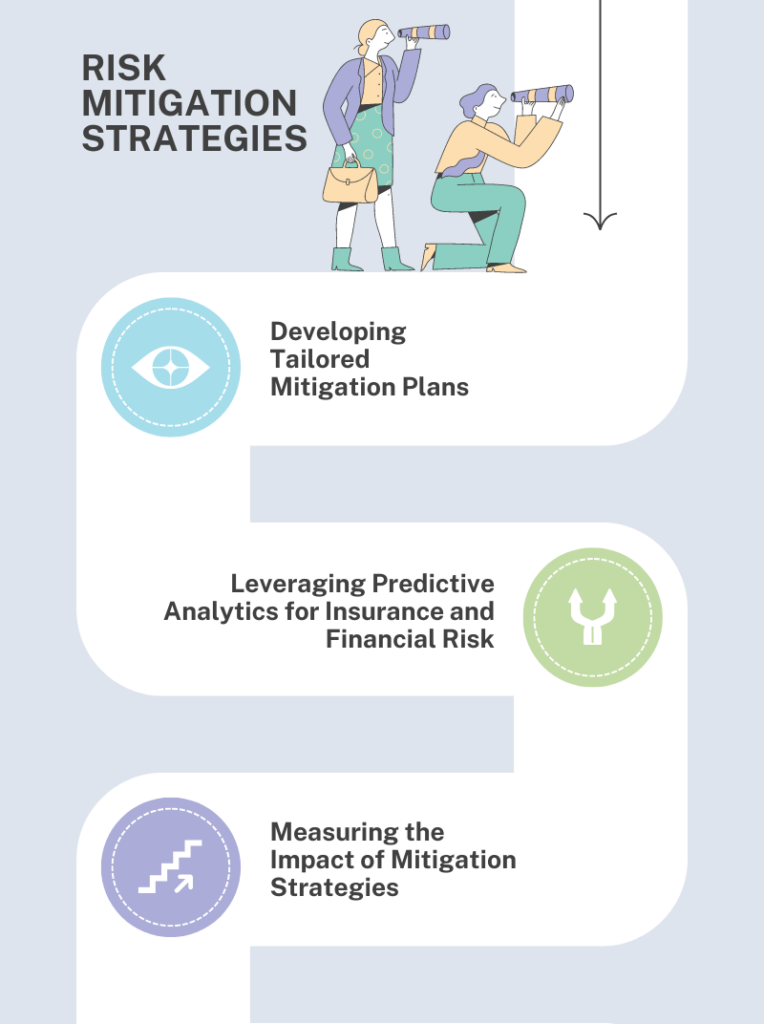
Developing Tailored Mitigation Plans
- Risk-Based Mitigation: By meticulously evaluating the likelihood and potential impact of diverse risks, organizations can strategically allocate resources to implement countermeasures.
- Scenario Planning: Predictive analytics can be used to simulate different scenarios and assess the effectiveness of potential mitigation strategies. This helps organizations develop robust contingency plans.
- Continuous Monitoring and Adjustment: The risk landscape is dynamic. Predictive models should be continuously monitored to detect changes in risk profiles. Mitigation plans must be adaptable to accommodate evolving threats.
Leveraging Predictive Analytics for Insurance and Financial Risk
- Insurance Underwriting: Predictive analytics can help insurers assess risk more accurately, leading to optimized pricing and underwriting decisions. By identifying high-risk customers, insurers can implement appropriate risk management measures.
- Fraud Detection: Predictive models can detect patterns indicative of fraudulent activities, enabling insurers to prevent losses and protect customers.
- Investment Risk Management: Predictive analytics empowers financial institutions to make data-driven investment decisions and manage portfolio risk effectively by analyzing market trends and economic indicators.
Measuring the Impact of Mitigation Strategies
To evaluate risk mitigation effectiveness, establish KPIs that measure risk reduction, score improvement, and overall business impact. Utilize predictive analytics to track these metrics and make data-driven adjustments.
By combining predictive analytics with effective risk mitigation strategies, organizations can significantly enhance their resilience and protect their bottom line.
Challenges and Limitations
Realizing the full potential of predictive analytics requires overcoming significant hurdles. Challenges such as data quality issues, model complexity, organizational resistance, and ongoing maintenance can hinder successful implementation. Addressing these obstacles is essential to unlocking the technology’s true value.
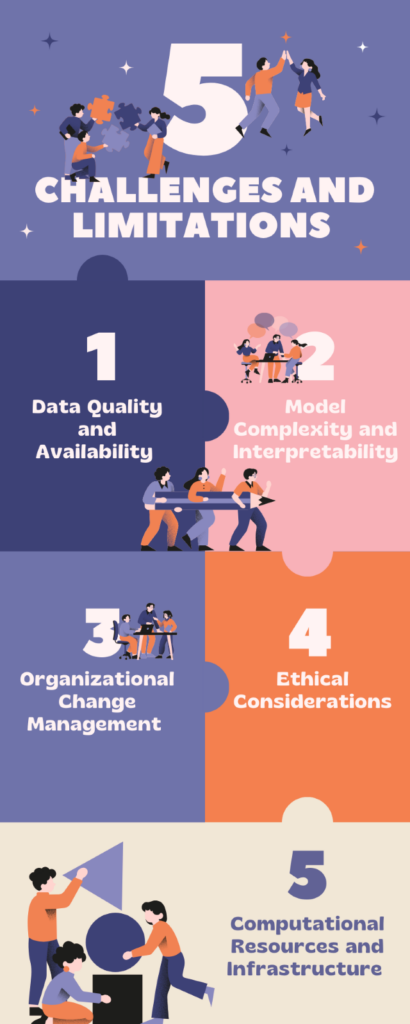
Data Quality and Availability
High-quality data is the cornerstone of effective predictive analytics. Addressing data inconsistencies, missing values, and biases is crucial for building reliable models. Organizations must prioritize robust data management practices to ensure data accuracy and completeness, ultimately driving better decision-making.
Model Complexity and Interpretability
Deep neural networks excel in achieving high accuracy but often lack transparency. Understanding the decision-making process of these complex models is crucial for building trust and ensuring responsible AI. The development of more interpretable models and explainable AI techniques is an ongoing area of research.
Computational Resources and Infrastructure
Predictive analytics, particularly when applied to large datasets, demands substantial computational resources. Organizations often require specialized hardware or cloud-based solutions to handle the intensive processing requirements associated with these models.
Organizational Change Management
Successful implementation of predictive analytics necessitates a cultural transformation within organizations. Overcoming resistance to change and fostering a data-driven culture are crucial steps in realizing the full potential of this technology.
Ethical Considerations
The use of data for predictive analytics raises ethical concerns, such as privacy, bias, and fairness. Organizations must ensure that their models are developed and used ethically, respecting individual rights and avoiding discriminatory outcomes.
Despite these challenges, the potential benefits of predictive analytics in risk management far outweigh the obstacles. By addressing these issues proactively, organizations can unlock the full value of this powerful technology.
Future Trends
The landscape of predictive analytics is continuously evolving, driven by advancements in technology and the growing complexity of risks. Several trends are shaping the future of risk management:
Integration of Artificial Intelligence and Machine Learning
AI and machine learning are set to revolutionize predictive analytics. These technologies will enable more complex models, improved accuracy, and the ability to handle vast amounts of data.
Real-Time Analytics and Predictive Modeling
The demand for real-time insights is increasing. Predictive models capable of processing and analyzing data in real-time will become essential for managing dynamic risks.
Explainable AI and Model Interpretability
As the complexity of models grows, the need for transparency increases. Explainable AI techniques will be crucial for understanding the rationale behind predictions, building trust, and meeting regulatory requirements.
Advanced Simulation and Scenario Planning
Predictive analytics will be increasingly used for complex scenario planning, allowing organizations to assess the impact of multiple variables on risk outcomes. This will enable more robust risk mitigation strategies.
Ethical Considerations and Bias Mitigation
As predictive analytics becomes more prevalent, addressing ethical concerns will be paramount. Organizations must focus on developing fair and unbiased models to avoid discriminatory outcomes.
Quantum Computing and Predictive Analytics
While still in its nascent stages, quantum computing has the potential to revolutionize predictive analytics by solving complex problems that are intractable for classical computers. This could lead to significant breakthroughs in risk management.
By staying informed about these trends and investing in research and development, organizations can harness the full potential of predictive analytics to build a resilient and future-proof risk management framework.
Conclusion
Predictive analytics is no longer a futuristic concept; it’s a strategic imperative for organizations seeking to thrive in an increasingly complex and uncertain world. By harnessing the power of data and advanced analytics, businesses can move from reactive to proactive risk management, safeguarding their assets and reputation.
From identifying potential threats to developing effective mitigation strategies, predictive analytics offers a holistic approach to risk management. While challenges such as data quality and model interpretability exist, the potential benefits far outweigh the obstacles.
As technology continues to evolve, so too will the capabilities of predictive analytics. By staying at the forefront of these advancements and adopting a data-driven culture, organizations can build a resilient and future-proof risk management framework.
At Codersperhour, we specialize in helping businesses harness the power of predictive analytics to transform their risk management practices. Our expertise, combined with cutting-edge technology, enables us to deliver tailored solutions that drive tangible results.
By partnering with Codersperhour, you can unlock the full potential of predictive analytics and gain a competitive edge in today’s dynamic business landscape.